Long, arduous root cause analysis investigations don’t lead to process improvements. Siloed data from machine sensor signals that take a data scientist to analyze and untangle. Persistent quality issues that frankly, there isn’t enough time to solve. And worst of all... the threat of a major quality spill looming around the corner.
These are the nightmares of manufacturing and quality engineers and managers.
Industry 4.0 has ushered in a wave of incredible advances to make manufacturing smarter, faster, and more precise. But quality teams have still been struggling with the same problems that they have for decades.
Now, advances in quality are finally available to change that.
What makes predictive quality possible?
Predictive quality is the term used to describe the new wave of advancement in quality that, when applied effectively, can drastically reduce scrap and rework, accelerate root cause analysis, and prevent quality spills. There are three technologies that predictive quality relies on to make this possible:
- AI and machine learning. AI and machine learning is now accessible and can be operationalized for a wide range of industrial applications. Talented engineers and data scientists have begun to explore different applications to solve problems in manufacturing. Recently, predictive quality tools that use machine learning have become commercially available.
- The maturity of data collection. Most manufacturers now have enough infrastructure in place and literacy around the data that their production environments generate. The availability of this data is crucial for predictive quality. Machine learning algorithms ingest this data and analyze it to solve quality problems.
- The adoption of cloud computing. Complex machine learning algorithms and large volumes of data take a tremendous amount of processing power. Cloud computing offers a fast, secure, and scalable platform on which a predictive quality tool can run.
The key to leveraging these three technologies to improve quality is to create an operational software platform that can be used to apply them on the shop floor. Speed is critical to manufacturing, and any new tool needs to keep up with the pace. A predictive quality tool can ingest and analyze data in real time. Engineers and operators can access the platform online to view data visualizations and analysis. All of their manufacturing data is in one place, to view in real time, or explore historical trends.

What Does Predictive Quality Actually Do?
To summarize so far, predictive quality helps manufacturers harness the growing breadth of production data through a combination of powerful cloud technologies and purpose-built AI and ML algorithms that derive insights not otherwise possible through time consuming manual means alone. A predictive quality tool is a digital platform that is designed for engineers and operators to help them solve production problems. A predictive quality tool focuses on three major functions:
• Connecting all manufacturing data. A predictive quality tool ingests process data, test data, and any other source that may influence product quality. Want to know how the ambient temperature of your facility affects the test performance of your products? Are you curious how a tolerance adjustment in operation 3 affects end-of-line test outcomes? You can discover the answers to these questions using a predictive quality tool easily. No more manual analysis using spreadsheets.
• Predicting quality issues on the line. In a predictive quality tool, machine learning algorithms ingest manufacturing and test data to deeply understand complex processes. The software alerts engineers and operators about upcoming production issues so that they can act swiftly to prevent them. Manufacturers no longer need to wait until end-of-line tests to discover defects.
• Automating root cause analysis investigations. The deep analysis and understanding that the predictive quality platform forms through analyzing hundreds or thousands of machine sensor signals makes it possible to show correlations between a product that failed a test, and the process conditions that created it. Engineers can instantly view a prioritized “short list” of sensor signals that relate to a defective part. This can save hours or days of manual investigation to find a problem.
Predictive quality is a shift left for manufacturing
The term “shift left” came from the software industry. It means to take the attention traditionally put towards quality testing at the end of the process and shift it “left”, as seen in the graph below. In software, this meant to start testing the quality of the code early on in the development process, instead of waiting until the end when a bug could be hard to fix at that point.
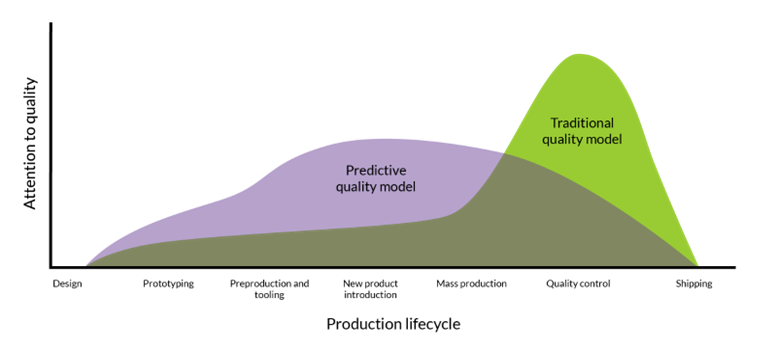
In manufacturing, shifting left refers to moving away from relying on quality gates such as end-of-line or in-process testing to find defects. Predictive quality allows manufacturers to spread the attention to quality more evenly throughout the process. By predicting defects before they happen, the process wastes much fewer resources and materials. Not only is quality improved, but so is overall efficiency in the plant.
Does predictive quality make statistical process control (SPC) obsolete?
Many manufacturers have a love-hate relationship with SPC. For many, this is the best tool they have to control quality and manage their process. But for some, the limitations of it are obvious. Readings from different sensor signals are siloed from one another, and unless they are all continuously monitored by a human, it is difficult to spot subtle trends. Understanding how these signals relate to one another and affect testing outcomes is an even greater challenge. Historical data often needs to be pulled from PLCs with a USB stick, and hours could be spent organizing and analyzing it to gain any insights.
Predictive quality has none of these limitations. It is flexible and adaptable, and can handle complex problems with ease. However, that doesn’t mean that SPC is going away. There is still a place for fixed control limits and control charts to work alongside machine learning algorithms in a predictive quality platform.
Dive deeper into predictive quality
This article only scratches the surface on the topic of predictive quality. Read the whitepaper authored by Greta Cutulenco, Mike Ciceri, Joseph Biron and Jim Ingerslew, a group of industry experts who have collaborated to define the term and detail its importance to manufacturers.
Sign up today for a free Essential Membership to Automation Alley to keep your finger on the pulse of digital transformation in Michigan and beyond.